Introduction
The Rise of Big Data in Healthcare
Using Big Data to Draw Inferences
Diagnosis and Monitoring
Applications of Data Analysis in Healthcare
Providers and Payers
Patients
Conclusion
References
Healthcare institutions produce large amounts of data in the form of electronic medical records, clinical trial records, billing, customer records management databases, etc. Scientific publications add huge amounts of information each second.
Such data is non-uniform and can be either structured or unstructured. Data that can be mined to produce invaluable insights into disease, prevention, and treatment is waiting to be recognized, processed, and used.
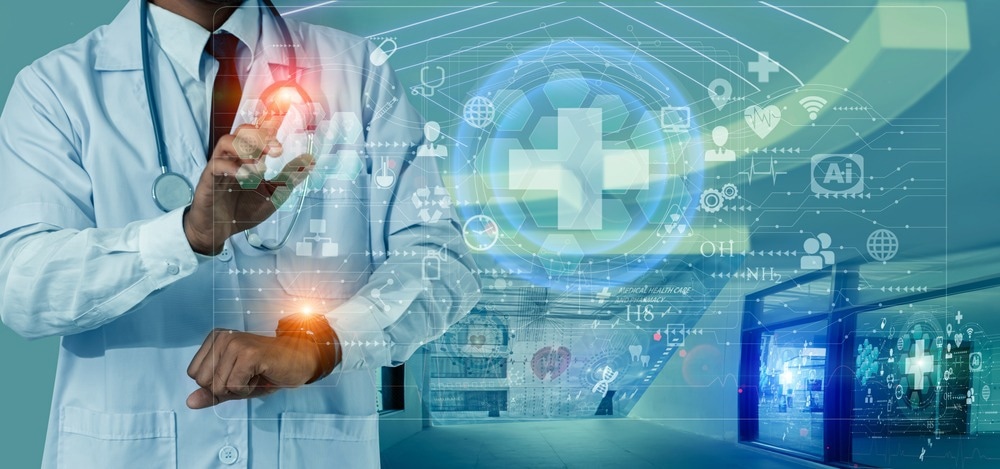
Image Credit: SOMKID THONGDEE/Shutterstock.com
Introduction
Data in healthcare can be pooled and analyzed to understand what patterns are evident and to analyze what drives those patterns so that preventive or therapeutic action can be taken as needed. This can help improve life expectancy or develop tests to detect and identify disease early, as well as provide treatment at a cost-effective level.
Health Information Exchange (HIE) is a mode of data utilization that can merge a patient's clinical information stored on the server of any medical facility into a single record that can be accessed by any of the patient's healthcare providers. To exploit the potential of this approach, sophisticated software programs, Artificial Intelligence (AI)-based algorithms, and high-end computers must be available to the healthcare organization to use and contribute to Big Data.
The Rise of Big Data in Healthcare
The acceleration in the availability of healthcare data is mainly due to the emergence of new devices, sophisticated testing technology, mobile applications, and wearables that provide some form of healthcare, whether in the diagnosis or the monitoring of disease. This has given rise to Big Data – "Big Data as "data that is so large, fast or complex that it's difficult or impossible to process using traditional methods."
Healthcare data sources include EMR, EHR, Web Service, Sensor Data, and Biometric Data. Government and patient portals, research studies, and generic databases also play a huge role.
Electronic health records (EHR) refer to the digital storage of medical information. This can now be done without manual data entry, using natural language processing (NLP) technology that recognizes and processes handwriting. This can be coupled with biometrics software to ensure that patient data is kept secure and to allow it to be accessed by authorized personnel at any point of need anywhere. The role of such technology in diagnosing and monitoring individual diseases is also being investigated and promises to deliver fast, accurate diagnoses.
Using Big Data to Draw Inferences
Big Data related to healthcare is valued at over 15 billion dollars globally and may increase by around 20% within a decade. New methods involving machine learning (ML) and AI are being implemented to gain valuable insights into disease.
ML refers to computer programs that analyze data with the help of dedicated algorithms that apply fuzzy logic and use neural networks. These programs help the machine self-adapt to changing data inputs without human inputs. The system thus learns to analyze the data to produce the desired output by using specific techniques and huge volumes of data.
Data science is now coming into its own in the healthcare field to enhance the effective and personalized implementation of medical care. Data mining refers to the various processes to which raw user information is subjected to extract useful conclusions. This can include data grouping, clustering, correlation, and searching for patterns in data, as well as regression and data storage.
The ML-aided analysis of medical images is among healthcare's most up-and-coming areas of data science. The ability to detect patterns and identify abnormalities via deep learning techniques has significantly contributed to the improved diagnosis of conditions using mammograms, X-rays, and other diagnostic imaging technologies, making them less dependent on observer error and inexperience.
Diagnosis and Monitoring
Again, similar technologies are very useful in developing sophisticated microbiologic diagnosis methods coupled with microbes' genomic data. Software programs that are able to recognize and differentiate the specific sequences and antigenic patterns of a microbe or part of a microbe can allow for the rapid diagnosis of infection without exposing humans to danger. Moreover, this automated process may reduce the possibility of human error.
Wearables allow data on the consumer's vital signs to be collected, along with multiple other parameters, including sleep, fluid intake, and mood changes. This enormous accumulation of data can be analyzed in real-time to monitor patients on follow-up and to detect departures from the normal as soon as they are identified for immediate reporting and action where necessary.
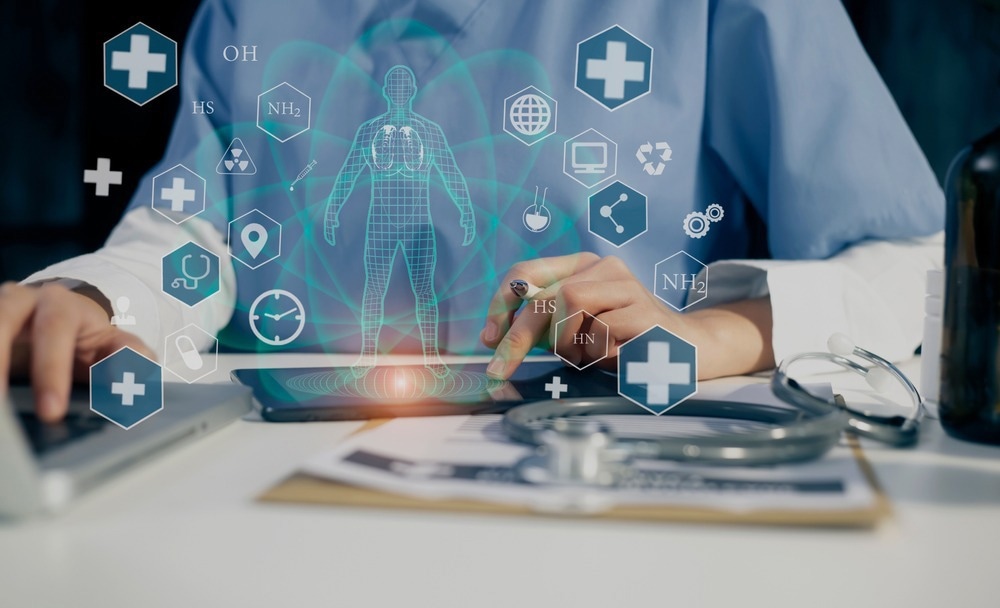
Image Credit: SOMKID THONGDEE/Shutterstock.com
Applications of Data Analysis in Healthcare
Providers and Payers
One area relates to healthcare providers, where data can be analyzed to help improve the efficiency of care and how well patients are shifted to their areas of care, in addition to ensuring that personalized care is obtained.
For healthcare payers like health insurance companies, data can drive applications to detect fraudulent claims but provide timely payments for genuine claims.
Patients
As for patients, they are the group that benefits the most from the proper use of data. Understanding disease and its causes, prevention, and treatment that comes from looking for patterns in medical data can improve the kind of treatment that is given, help tailor it for individual needs, and provide increased accuracy of diagnosis.
Using past data, combined with wearables, it is possible to track patients and identify rising biomarkers before a clinical event occurs. Analytic software can identify high-risk habits, predict the risk of specific health events, and suggest preventive plans. In addition, Big Data can help prevent and predict larger outbreaks.
Again, pharmaceuticals could benefit immensely from the use of data. Considering the mean cost of successful drug development, at over $2.5 billion, and the timeline of at least a decade, the advantages of using Big Data are obvious. The analysis of Big Data helps identify the drugs that are needed most.
The ability to search for and examine previous papers, patents and trials can uncover mechanisms of drug action on the body and may help correlate different events. These insights could speed up the development of new drugs built on previous research, using algorithms to, for instance, simulate the drug action in the human body or using real-world data to simulate a clinical trial.
Marketing and sales strategizing could also gain much from analyzing earlier data. And importantly, pharmacovigilance, which involves tracking and identifying adverse drug reactions post-marketing, can be carried out much better using adverse event reports (AERs).
Conclusion
Overall, data appears to be at the forefront of medical advances to streamline services, improve diagnostic accuracy and propel new drug discoveries into needed channels while saving time and money. Epidemic prevention and control is also an area that can benefit hugely from data-driven operations.
Many challenges exist, such as data disorganization or inaccuracy, affecting the integrity of the data, patient safety without restricting data quality and accessibility, data standardization, language barriers, and incorporating genomic data.
Continue Reading: Unlocking the Power of Data to Accelerate Future Health Systems
References:
Further Reading